Coronary artery disease is the top cause of death worldwide. A common way to diagnose and monitor heart issues is through myocardial perfusion imaging (MPI) using single photon emission computed tomography (SPECT). This process involves using a radioactive tracer and specialized camera to create detailed images of blood flow to the heart. It helps doctors spot coronary artery disease and other heart problems. However, standard SPECT imaging needs an additional CT scan for precise results, which means more radiation for patients and higher costs.
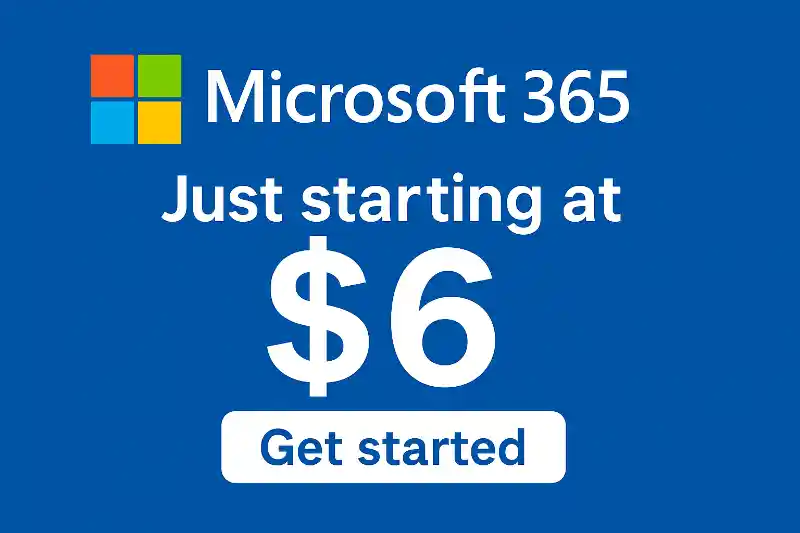
Researchers at Washington University in St. Louis, along with experts from Cleveland Clinic and the University of California Santa Barbara, are working on an exciting new method called CTLESS. This innovative technique uses deep learning to eliminate the need for a CT scan without affecting diagnostic quality. The study led by Abhinav Jha, an associate professor in biomedical engineering and radiology, was published on November 25 in IEEE Transactions in Medical Imaging.
The next step for the team is to further validate this method. They aim to make it more accessible, especially in rural hospitals where such technology may be hard to come by. This could be a game changer for areas with limited access to advanced imaging.
In traditional SPECT imaging, a CT scan is needed to correct for how the emitted signals weaken as they pass through body tissue. Without this correction, heart images can be unclear or misleading. Unfortunately, about 75% of SPECT MPI scans are done without this critical correction due to costs, complex equipment needs, and other local issues. The CTLESS method addresses this by creating a synthetic attenuation map to improve image quality without needing a CT scan.
“Many hospitals don’t have the equipment for a CT scan, which can affect the accuracy of heart imaging. CTLESS offers a way to get reliable results without an extra scan,”
Abhinav Jha, associate professor of biomedical engineering and radiology
CTLESS enhances image clarity by using information from the SPECT scan itself. The research team tested CTLESS with real clinical data and found it performed similarly to traditional methods. It worked well across different scanner types, varying levels of heart damage, and diverse patient groups. Notably, it performed reliably for both men and women, demonstrating that it can adapt to anatomical differences in both sexes.
Jha mentioned, “Our findings suggest that in the future, we might not need a CT scan for attenuation correction in MPI SPECT. This is especially important for patients in rural areas where such scans are not easily accessible.” By reducing the need for an extra scan, CTLESS can help improve health care equality both in the U.S. and globally.
This research was partially funded by the National Institute of Biomedical Imaging and Bioengineering and the National Science Foundation, among others. For a deeper dive into their findings, check out the publication: CTLESS: A scatter-window projection and deep learning-based transmission-less attenuation compensation method for myocardial perfusion SPECT in IEEE Transactions in Medical Imaging. You can find it at doi.org/10.1109/TMI.2024.3496870.
Source link
Deep Learning, Heart, Imaging, Blood, Computed Tomography, Coronary Artery Disease, CT, Diagnostic, Heart Disease, Medical Imaging, Medicine, Radiology, Research, Tomography